7 Critical Factors in Choosing Between Off-the-Shelf AI Product and Custom AI Solutions
16.04.2025 | 12 min read
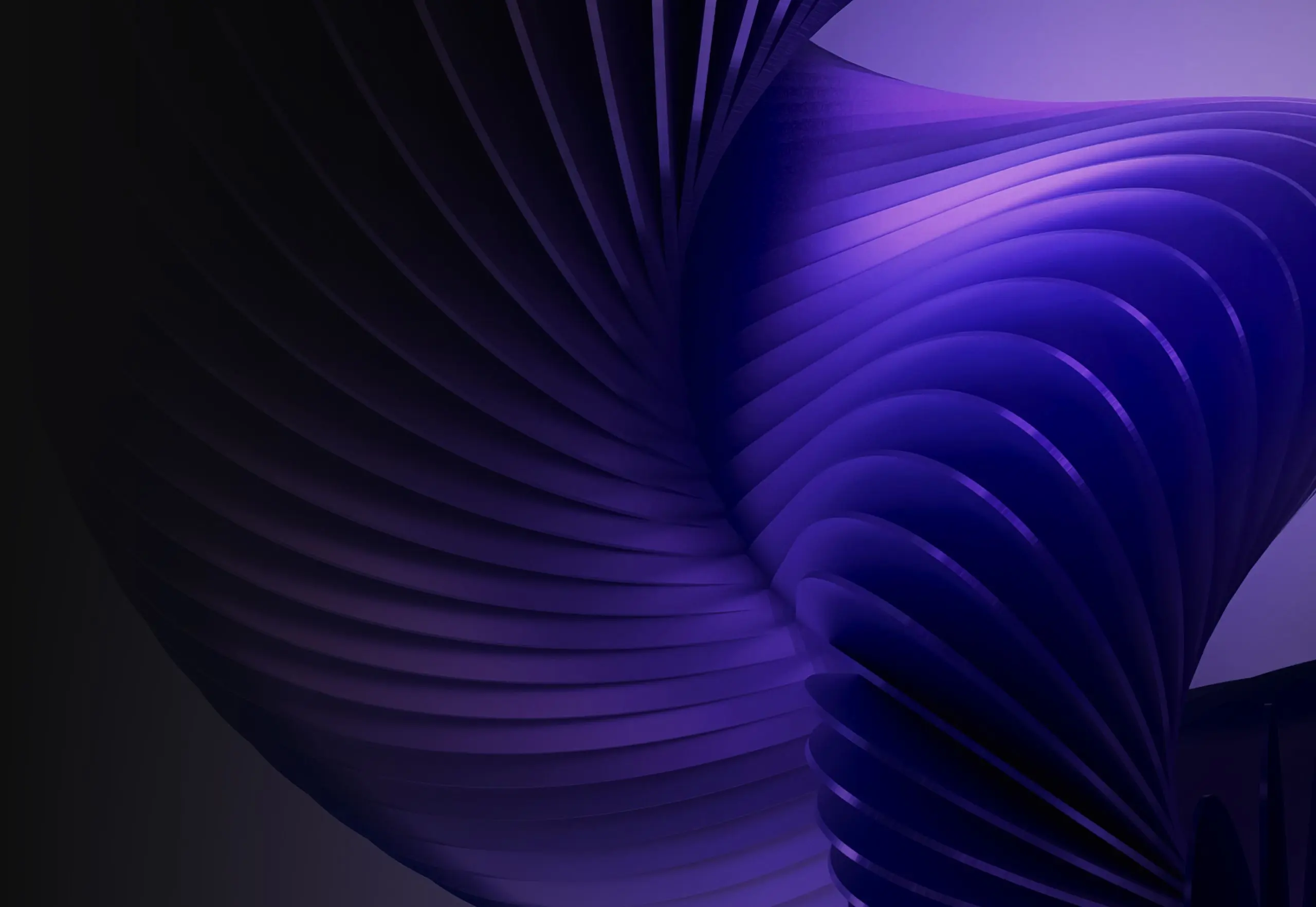
Are you standing at the AI crossroads, wondering which path will best serve your business needs? You're not alone. As Generative AI transforms industries at breakneck speed, companies face a pivotal decision: leverage existing off-the-shelf AI solutions or invest in a custom AI solution.
Let me guide you through this decision landscape with clarity and actionable insights on artificial intelligence implementation options.
1. Is Speed vs. Specificity What's Your AI Tool Priority?
Ready-made AI platforms like OpenAI's GPT or Google's Vertex AI offer an express lane to implementation. You can integrate AI tools in days or weeks, not months.
But this speed comes at a cost: genericity.
Off-the-shelf solutions deliver:
- Rapid deployment (days to weeks)
- Lower technical barriers to entry
- Immediate access to state-of-the-art AI models
Custom AI solutions through development partners like 10Clouds take longer but create precision-engineered AI systems tailored to your exact requirements, workflows, and proprietary data.
Is time your most precious resource right now? Or is a perfect-fit custom solution worth the wait? Your answer guides your first step in AI software development.
2. How Should You Analyze Upfront Investment vs. Long-Term Costs of Integrating AI?
The financial equation isn't as simple as "cheap vs. expensive" when exploring the pros and cons.
Ready-to-use AI solutions require minimal upfront investment. Many offer free tiers or credits for initial testing, with subscription-based or pay-as-you-go pricing models. This makes these off-the-shelf AI products incredibly accessible.
But watch out for the scaling trap.
As usage increases, those predictable per-token or per-call costs can balloon dramatically. What seemed cost-effective can become a significant operational expense when implementing AI solutions.
Custom AI development demands substantial initial capital—potentially $50,000 to $500,000 depending on complexity. Yet a custom AI solution may deliver better total cost of ownership (TCO) at scale over time, especially when factoring in the value of resulting intellectual property.
Consider your financial horizon: Are you optimizing for minimal initial outlay or maximum long-term value with AI capabilities?
3. Who Owns Your AI Model: Control & Security Considerations?
In today's data-sensitive environment, this factor alone might determine whether you choose custom AI solutions vs off-the-shelf options.
With ready-made AI software, you're sending potentially sensitive data to third parties. Your artificial intelligence capabilities are also constrained by the provider's decisions about model updates, pricing changes, and feature roadmaps.
Custom AI solutions offer full sovereignty over:
- Where and how your data is stored
- Which data trains your models
- The deployment environment (cloud, on-premise, hybrid)
- The intellectual property created
For regulated industries or organizations handling sensitive information, this control can be non-negotiable. The bespoke AI approach ensures that the AI system respects your specific security requirements.
4. How Will You Achieve Competitive Differentiation?
Here's a stark truth: If you and your competitors use identical off-the-shelf AI tools, how will you differentiate?
Ready-made AI platforms provide powerful capabilities, but they're available to everyone. The same API powering your chatbot might be running your competitor's identical feature.
Custom solutions are designed to create truly novel capabilities that competitors can't easily replicate. This competitive moat becomes increasingly valuable as artificial intelligence shifts from novelty to necessity.
Ask yourself: Is this AI software a core differentiator for my business or simply a supporting function? Pre-built AI solutions may be sufficient for standard operations, but tailored AI solutions offer unique advantages for your core business processes.
5. Should You Prioritize Superficial or Seamless Integration?
How deeply must your AI solution connect with existing systems?
Off-the-shelf software often integrates through standardized APIs—sufficient for many applications but potentially limiting for complex environments.
Custom AI solutions typically require more effort upfront but are designed from the ground up with your specific technology ecosystem in mind. They can achieve deeper integration with your databases, enterprise software, and operational workflows.
10Clouds, for example, emphasizes building custom software development solutions that increase ROI by integrating AI seamlessly with existing systems—not just adding an artificial intelligence layer on top.
6. How Will You Balance Internal Capabilities vs. AI Development Partner Reliance?
Your internal technical capabilities should heavily influence your choice between custom and off-the-shelf AI development.
Ready-to-use solutions require varying levels of technical expertise:
- SaaS tools often need minimal technical knowledge
- API integration requires software development skills
- Fine-tuning needs some machine learning understanding
Custom AI development partnerships demand different abilities:
- Project management expertise
- Domain knowledge to guide requirements
- Technical oversight capacity
With limited internal AI expertise, you'll rely heavily on your personalized AI solutions partner for custom projects. This creates a different kind of dependency than vendor lock-in but requires careful relationship management.
7. Is A Hybrid Approach The Best Solution For Your Business Needs?
The good news? This isn't necessarily an either/or decision between custom AI vs off-the-shelf solutions.
Many organizations successfully adopt a portfolio approach—using ready-made AI solutions for standard functions while investing in custom AI software development for strategically critical applications.
Consider starting with off-the-shelf AI products to:
- Build initial understanding of AI technologies
- Demonstrate quick wins and ROI
- Identify high-value applications worth custom investment
Then partner with an AI development company for custom development where it delivers maximum strategic value.
What Are The Ready-Made AI Platform Options You Should Know?
Before deciding, you need to understand what's actually available in the marketplace. The "ready-made" ecosystem is vast and rapidly evolving.
Foundational Model APIs: These provide direct access to powerful, general-purpose AI models.
- OpenAI (GPT-4, DALL-E) - The market leader with robust text and image generation
- Google Vertex AI (Gemini models) - Comprehensive suite with multimodal capabilities
- Anthropic Claude - Known for safety, reliability, and ethical considerations
- Amazon Bedrock - Access to multiple foundation models in a single platform
Specialist APIs: These focus on specific AI capabilities with exceptional performance.
- ElevenLabs - Specializing in ultra-realistic voice synthesis
- Stability AI - Leaders in image generation with Stable Diffusion
- Cohere - Focusing on enterprise-grade text generation and embedding
- Groq - Offering extremely fast inference speeds for real-time applications
Off-the-shelf AI products: These are ready-to-use applications with embedded artificial intelligence capabilities.
- Content Creation: Jasper, Writer, Anyword
- Design: Midjourney, Adobe Firefly, Canva AI
- Customer Support: Zapier Chatbots, Chatbase, Aisera
- Sales: Pitch (for sales decks), Salesforce Einstein GPT
Middleware & Aggregators: These platforms help manage multiple AI models.
- LangChain - Framework for creating LLM-powered applications
- Lamatic, Eden AI, OpenRouter - Providing unified access to multiple models
What's the takeaway? The ready-made AI landscape offers specialized tools for nearly every business function. Understanding these categories helps you identify where generic AI solutions may suffice and where developing a custom AI solution delivers unique value.
How Do These Approaches Compare In Real-World Implementation?
Let's see how these approaches play out in a concrete business scenario: implementing an AI-powered customer service solution for a mid-sized e-commerce company.
Ready-Made Approach:
- Solution: Integrating OpenAI's GPT-4 API into existing ticketing system + fine-tuning on FAQ data
- Timeline: 3-4 weeks for integration and testing
- Initial Cost: ~$15,000 for development work + $0.03-0.06 per query
- Monthly Cost (10,000 queries): ~$300-600 in API fees
- Control: Limited to prompt engineering and some fine-tuning
- Data Handling: Customer queries processed via third-party AI solutions
- Maintenance: Minimal; primarily monitoring API usage and costs
Custom Development Approach:
- Solution: Bespoke AI solutions developed with a custom AI development partner, including domain-specific training
- Timeline: 4-5 months for development and deployment
- Initial Cost: ~$150,000-250,000 for full custom AI software development
- Monthly Cost: ~$5,000 for hosting and maintenance
- Control: Complete ownership of custom models, data processing, and deployment
- Data Handling: All data processed in owned/controlled environments
- Maintenance: Ongoing partnership for model improvements and feature additions
The Results Comparison:
- Accuracy: Custom AI solution achieves 15-20% higher accuracy on company-specific queries
- Customer Satisfaction: Custom AI provides more consistent brand voice and handling of complex issues
- Scalability: Ready-made AI tool costs increase linearly with usage; custom solution costs remain relatively stable
- Long-term ROI: Break-even point at approximately 24 months for the custom AI solution
Real-world lesson: For standardized customer service needs with moderate volume, off-the-shelf solutions are developed to offer an attractive cost-benefit ratio. For companies with complex products, high query volumes, or stringent data requirements, custom AI solutions provide superior long-term value despite higher initial investment.
What Technical Considerations Go Beyond The Business Case?
The technical architecture of your artificial intelligence solution will significantly impact its long-term success. Here are critical considerations beyond pure business factors:
Model Architecture Choices:
- Large Language Models (LLMs) - Best for text generation, reasoning, and conversation
- Multimodal Models - Combining text, image, audio capabilities
- Smaller Specialized Models - More efficient for narrower tasks
Data Processing Approaches:
- Fine-tuning - Adapting existing AI models to your specific data
- Retrieval-Augmented Generation (RAG) - Combining LLMs with your knowledge base
- Training from scratch - Building domain-specific models (rare and expensive)
Deployment Options:
- Cloud-based - Maximum flexibility, minimal infrastructure management
- On-premises - Maximum data security, higher maintenance requirements
- Hybrid - Balancing security and ease of management
Integration Methods:
- API-first design - Enabling maximum interoperability
- Microservices architecture - Creating modular, scalable systems
- Event-driven processing - Managing asynchronous workflows efficiently
Technical insight: Many custom-built solutions don't build completely new models but rather construct sophisticated architectures around existing open-source models. The value often lies in the unique combination of components, data processing pipelines, and integration methods.
10Clouds, for example, has demonstrated expertise in creating RAG implementations that allow companies to leverage powerful foundation models while keeping proprietary data secure and maintaining query relevance.
How Can You Decide Between Custom AI Software or Off-the-Shelf AI Solution?
To help you make this critical decision, here's a practical framework you can apply to your specific situation:
Step 1: Score Your Strategic Factors (1-5 scale)
7 Critical Factors in Choosing Between Off-the-Shelf AI and Custom AI Solutions
Are you standing at the AI crossroads, wondering which path will best serve your business needs? You're not alone. As Generative AI transforms industries at breakneck speed, companies face a pivotal decision: leverage existing off-the-shelf AI solutions or invest in a custom AI solution.
Let me guide you through this decision landscape with clarity and actionable insights on artificial intelligence implementation options.
Is Speed vs. Specificity What's Your Priority?
Ready-made AI platforms like OpenAI's GPT or Google's Vertex AI offer an express lane to implementation. You can integrate AI tools in days or weeks, not months.
But this speed comes at a cost: genericity.
Off-the-shelf solutions deliver:
- Rapid deployment (days to weeks)
- Lower technical barriers to entry
- Immediate access to state-of-the-art AI models
Custom AI solutions through development partners like 10Clouds take longer but create precision-engineered AI systems tailored to your exact requirements, workflows, and proprietary data.
Is time your most precious resource right now? Or is a perfect-fit custom solution worth the wait? Your answer guides your first step in AI software development.
How Should You Analyze Upfront Investment vs. Long-Term Costs?
The financial equation isn't as simple as "cheap vs. expensive" when exploring the pros and cons.
Ready-to-use AI solutions require minimal upfront investment. Many offer free tiers or credits for initial testing, with subscription-based or pay-as-you-go pricing models. This makes these off-the-shelf AI products incredibly accessible.
But watch out for the scaling trap.
As usage increases, those predictable per-token or per-call costs can balloon dramatically. What seemed cost-effective can become a significant operational expense when implementing AI solutions.
Custom AI development demands substantial initial capital—potentially $50,000 to $500,000 depending on complexity. Yet a custom AI solution may deliver better total cost of ownership (TCO) at scale over time, especially when factoring in the value of resulting intellectual property.
Consider your financial horizon: Are you optimizing for minimal initial outlay or maximum long-term value with AI capabilities?
Who Owns Your AI Future: Control & Security Considerations?
In today's data-sensitive environment, this factor alone might determine whether you choose custom AI solutions vs off-the-shelf options.
With ready-made AI software, you're sending potentially sensitive data to third parties. Your artificial intelligence capabilities are also constrained by the provider's decisions about model updates, pricing changes, and feature roadmaps.
Custom AI solutions offer full sovereignty over:
- Where and how your data is stored
- Which data trains your models
- The deployment environment (cloud, on-premise, hybrid)
- The intellectual property created
For regulated industries or organizations handling sensitive information, this control can be non-negotiable. The bespoke AI approach ensures that the AI system respects your specific security requirements.
How Will You Achieve Competitive Differentiation?
Here's a stark truth: If you and your competitors use identical off-the-shelf AI tools, how will you differentiate?
Ready-made AI platforms provide powerful capabilities, but they're available to everyone. The same API powering your chatbot might be running your competitor's identical feature.
Custom solutions are designed to create truly novel capabilities that competitors can't easily replicate. This competitive moat becomes increasingly valuable as artificial intelligence shifts from novelty to necessity.
Ask yourself: Is this AI software a core differentiator for my business or simply a supporting function? Pre-built AI solutions may be sufficient for standard operations, but tailored AI solutions offer unique advantages for your core business processes.
Should You Prioritize Superficial or Seamless Integration?
How deeply must your AI solution connect with existing systems?
Off-the-shelf AI software often integrates through standardized APIs—sufficient for many applications but potentially limiting for complex environments.
Custom AI solutions typically require more effort upfront but are designed from the ground up with your specific technology ecosystem in mind. They can achieve deeper integration with your databases, enterprise software, and operational workflows.
10Clouds, for example, emphasizes building custom software development solutions that increase ROI by integrating AI seamlessly with existing systems—not just adding an artificial intelligence layer on top.
How Will You Balance Internal Capabilities vs. Partner Reliance?
Your internal technical capabilities should heavily influence your choice between custom and off-the-shelf AI development.
Ready-to-use solutions require varying levels of technical expertise:
- SaaS tools often need minimal technical knowledge
- API integration requires software development skills
- Fine-tuning needs some machine learning understanding
Custom AI development partnerships demand different abilities:
- Project management expertise
- Domain knowledge to guide requirements
- Technical oversight capacity
With limited internal AI expertise, you'll rely heavily on your custom AI development partner for custom projects. This creates a different kind of dependency than vendor lock-in but requires careful relationship management.
Is A Hybrid Approach The Best Solution?
The good news? This isn't necessarily an either/or decision between custom AI vs off-the-shelf solutions.
Many organizations successfully adopt a portfolio approach—using ready-made AI solutions for standard functions while investing in custom AI software development for strategically critical applications.
Consider starting with off-the-shelf AI products to:
- Build initial understanding of AI technologies
- Demonstrate quick wins and ROI
- Identify high-value applications worth custom investment
Then partner with an AI development company for custom development where it delivers maximum strategic value.
What Are The Ready-Made Platform Options You Should Know?
Before deciding, you need to understand what's actually available in the marketplace. The "ready-made" ecosystem is vast and rapidly evolving.
Foundational Model APIs: These provide direct access to powerful, general-purpose AI models.
- OpenAI (GPT-4, DALL-E) - The market leader with robust text and image generation
- Google Vertex AI (Gemini models) - Comprehensive suite with multimodal capabilities
- Anthropic Claude - Known for safety, reliability, and ethical considerations
- Amazon Bedrock - Access to multiple foundation models in a single platform
Specialist APIs: These focus on specific AI capabilities with exceptional performance.
- ElevenLabs - Specializing in ultra-realistic voice synthesis
- Stability AI - Leaders in image generation with Stable Diffusion
- Cohere - Focusing on enterprise-grade text generation and embedding
- Groq - Offering extremely fast inference speeds for real-time applications
Off-the-shelf AI products: These are ready-to-use applications with embedded artificial intelligence capabilities.
- Content Creation: Jasper, Writer, Anyword
- Design: Midjourney, Adobe Firefly, Canva AI
- Customer Support: Zapier Chatbots, Chatbase, Aisera
- Sales: Pitch (for sales decks), Salesforce Einstein GPT
Middleware & Aggregators: These platforms help manage multiple AI models.
- LangChain - Framework for creating LLM-powered applications
- Lamatic, Eden AI, OpenRouter - Providing unified access to multiple models
What's the takeaway? The ready-made AI landscape offers specialized tools for nearly every business function. Understanding these categories helps you identify where generic AI solutions may suffice and where developing a custom AI solution delivers unique value.
How Do These Approaches Compare In Real-World Implementation?
Let's see how these approaches play out in a concrete business scenario: implementing an AI-powered customer service solution for a mid-sized e-commerce company.
Ready-Made Approach:
- Solution: Integrating OpenAI's GPT-4 API into existing ticketing system + fine-tuning on FAQ data
- Timeline: 3-4 weeks for integration and testing
- Initial Cost: ~$15,000 for development work + $0.03-0.06 per query
- Monthly Cost (10,000 queries): ~$300-600 in API fees
- Control: Limited to prompt engineering and some fine-tuning
- Data Handling: Customer queries processed via third-party AI solutions
- Maintenance: Minimal; primarily monitoring API usage and costs
Custom Development Approach:
- Solution: Bespoke AI solutions developed with a custom AI development partner, including domain-specific training
- Timeline: 4-5 months for development and deployment
- Initial Cost: ~$150,000-250,000 for full custom AI software development
- Monthly Cost: ~$5,000 for hosting and maintenance
- Control: Complete ownership of custom models, data processing, and deployment
- Data Handling: All data processed in owned/controlled environments
- Maintenance: Ongoing partnership for model improvements and feature additions
The Results Comparison:
- Accuracy: Custom AI solution achieves 15-20% higher accuracy on company-specific queries
- Customer Satisfaction: Custom AI provides more consistent brand voice and handling of complex issues
- Scalability: Ready-made AI tool costs increase linearly with usage; custom solution costs remain relatively stable
- Long-term ROI: Break-even point at approximately 24 months for the custom AI solution
Real-world lesson: For standardized customer service needs with moderate volume, off-the-shelf solutions are developed to offer an attractive cost-benefit ratio. For companies with complex products, high query volumes, or stringent data requirements, custom AI solutions provide superior long-term value despite higher initial investment.
What Technical Considerations Go Beyond The Business Case?
The technical architecture of your artificial intelligence solution will significantly impact its long-term success. Here are critical considerations beyond pure business factors:
Model Architecture Choices:
- Large Language Models (LLMs) - Best for text generation, reasoning, and conversation
- Multimodal Models - Combining text, image, audio capabilities
- Smaller Specialized Models - More efficient for narrower tasks
Data Processing Approaches:
- Fine-tuning - Adapting existing AI models to your specific data
- Retrieval-Augmented Generation (RAG) - Combining LLMs with your knowledge base
- Training from scratch - Building domain-specific models (rare and expensive)
Deployment Options:
- Cloud-based - Maximum flexibility, minimal infrastructure management
- On-premises - Maximum data security, higher maintenance requirements
- Hybrid - Balancing security and ease of management
Integration Methods:
- API-first design - Enabling maximum interoperability
- Microservices architecture - Creating modular, scalable systems
- Event-driven processing - Managing asynchronous workflows efficiently
Technical insight: Many custom-built solutions don't build completely new models but rather construct sophisticated architectures around existing open-source models. The value often lies in the unique combination of components, data processing pipelines, and integration methods.
10Clouds, for example, has demonstrated expertise in creating RAG implementations that allow companies to leverage powerful foundation models while keeping proprietary data secure and maintaining query relevance.
How Can You Decide Between Custom AI Solution or Off-the-Shelf AI Software?
To help you make this critical decision, here's a practical framework you can apply to your specific situation:
Step 1: Score Your Strategic Factors (1-5 scale)
Factor 1: Speed to implementation
- Weight (1-3): ___
- Ready-Made Score: ___
- Custom Score: ___
Factor 2: Budget constraints
- Weight (1-3): ___
- Ready-Made Score: ___
- Custom Score: ___
Factor 3: Data sensitivity
- Weight (1-3): ___
- Ready-Made Score: ___
- Custom Score: ___
Factor 4: Need for differentiation
- Weight (1-3): ___
- Ready-Made Score: ___
- Custom Score: ___
Factor 5: Internal technical expertise
- Weight (1-3): ___
- Ready-Made Score: ___
- Custom Score: ___
Factor 6: Scale of intended usage
- Weight (1-3): ___
- Ready-Made Score: ___
- Custom Score: ___
Factor 7: Integration complexity
- Weight (1-3): ___
- Ready-Made Score: ___
- Custom Score: ___
Step 2: Calculate Weighted Scores
Multiply each score by its weight, then total the columns to see which approach better matches your priorities.
Step 3: Ask These Critical Questions
- What's our true time horizon for this AI investment?
- How does this capability align with our competitive strategy?
- What's the worst-case scenario for data breaches or leakage?
- Do we have the right partner relationships for our preferred approach?
Step 4: Define Your Success Metrics
Before implementing AI solutions, clearly define what success looks like:
- Specific performance benchmarks
- Cost targets and ROI timeline
- User adoption rates
- Customer impact metrics
Pro tip: Many organizations benefit from starting with a Proof of Concept (PoC) project. Whether pursuing ready-made AI or developing AI solutions from scratch, a focused 4-8 week PoC can validate assumptions, demonstrate value, and reveal hidden challenges before full-scale implementation.
What Strategic Framework Should Guide Your Decision?
Now let's put this into action with clear recommendations for common scenarios:
For startups and SMBs with limited budgets: Start with off-the-shelf AI model with generous free/low-cost tiers for experimentation and quick wins.
For established companies with non-core AI needs: Choose enterprise-grade SaaS or well-supported APIs for standard functions where differentiation isn't critical.
For organizations with highly sensitive data or in regulated industries: Custom AI solutions are tailored to mitigate third-party risks.
For businesses where artificial intelligence capabilities are central to competitive advantage: Invest in a custom AI solution that creates unique intellectual property and proprietary capabilities.
For companies just beginning their AI journey: Start with ready-to-use AI products while building internal understanding, then strategically develop a custom AI solution for high-value applications.
Remember, even with custom AI development, you're not starting from zero. Partners like 10Clouds often leverage existing open-source models and middleware, customizing and extending them to create your unique AI solution. See best custom generative AI app development companies and top AI automation agencies.
The AI landscape continues evolving at unprecedented speed. Today's decision between custom and ready-made AI isn't permanent—but it will shape your organization's relationship with this transformative technology for years to come.
What's your next move at the AI crossroads? Will you build a custom AI solution or use an off-the-shelf AI product?