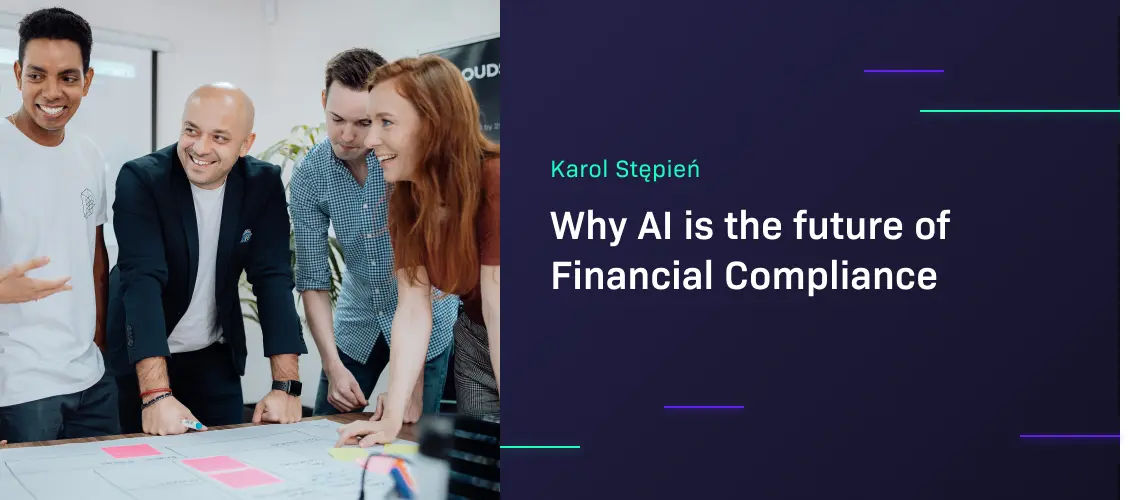
AI has been evolving at an unprecedented speed over the past decade, and the financial sector in particular has seen a recent rise in the number of adopted ML solutions. Many banks and FinTechs are now asking the question of what the future holds when it comes to AI and compliance. There are no doubt many benefits, including increased transaction efficiency and reduced customer friction, but can machine learning be entrusted with such an important task? We investigate this vital element of regulatory technology (RegTech).
An introduction to AI and Compliance
Before we delve deeper into the problems that AI can solve when it comes to compliance, let’s go through what the traditional role of a compliance team in a banking or financial institution involves - namely, making sure that their employer operates with integrity and adheres to applicable laws, regulations and internal policies.
Most banks and FinTechs have a compliance mechanism which is rule-based, and flags up any outliers based on set transaction thresholds. Compliance personnel would review these to spot any anomalies or trends. They also make adjustments to the thresholds based on their bank or FinTech’s risk tolerance. Between them, the team usually has many years of experience and expertise.
So what are the downsides? Well, relying solely on team expertise is risky, as humans are fallible and there is always a chance of them missing less common or frequent cases. There’s also the issue of false positives, as rule-based systems produce alerts based on transactional value, and a very high percentage of them are false positives. In fact when it comes to anti-money laundering, Fortytwo Data calculates that the cost of false positives amounts to 42% of the total outlay on companies’ AML compliance, which equals around £2.7bn. Here’s where machine learning can play a big part in improving efficiency and therefore reducing costs.
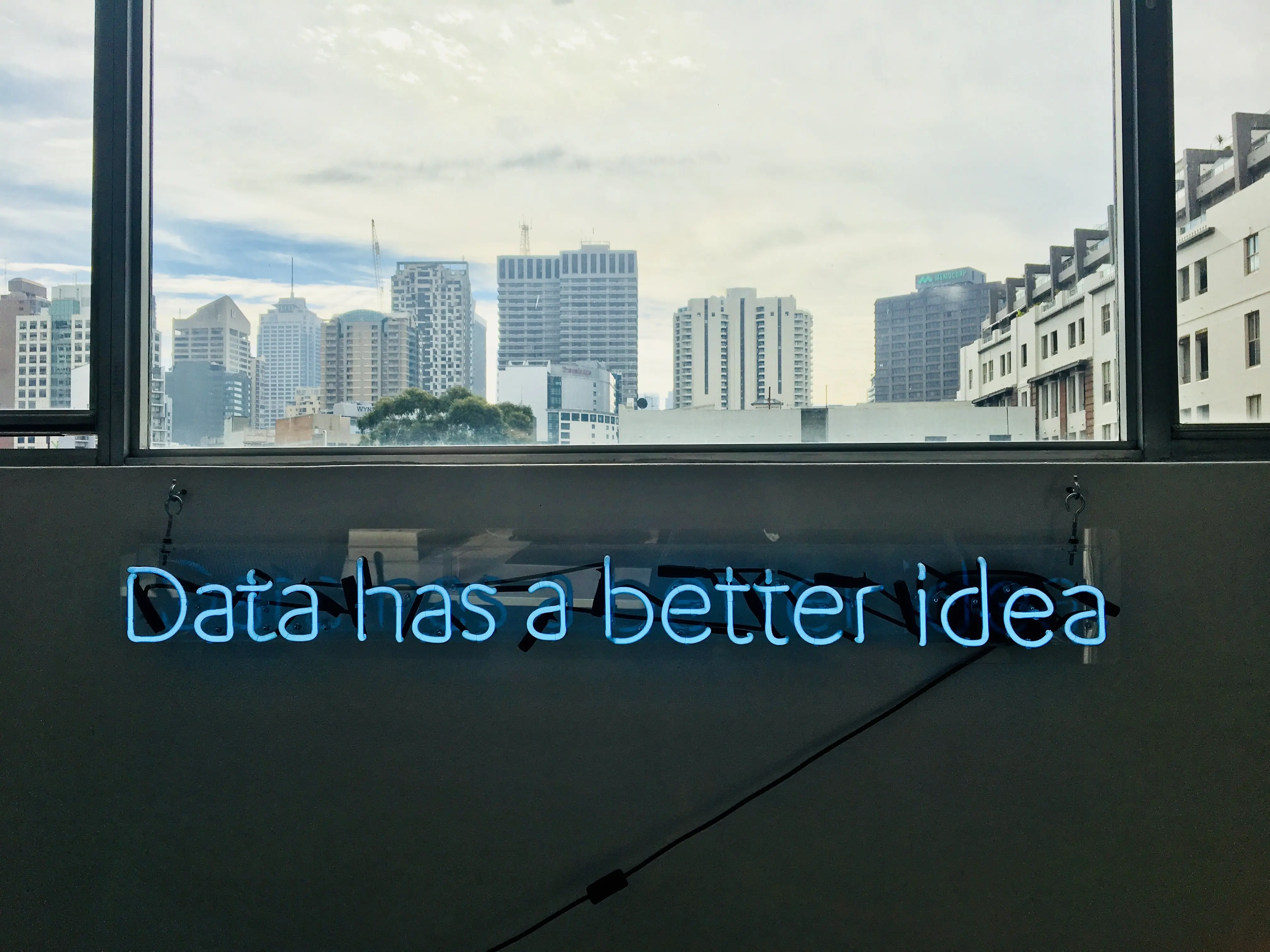
AI as the key to reducing false alerts
Machine learning algorithms allow banks and FinTechs to analyze AML alerts with greater speed and accuracy than their human counterparts. They enable the fast performance of semantic and statistical analyses and can be taught to efficiently identify redundant data and to remove duplicates, both of which lead to false positives. In addition, machine learning models can be built to create intuitive screening processes which remove the likelihood of confusing naming conventions or other set-up issues that could be generating false alerts.
Banks and FinTechs can train supervised machine learning algorithms on existing alerts to allow for the fine-tuning of thresholds. AI can compare current rules with the results of previous investigations and use this to inform future rule-setting. Similarly, unsupervised algorithms can be used to identify new cases without the help of rules.
All this means that the financial institution can streamline the alert remediation process by immediately prioritizing particular cases and bringing them to the attention of the compliance team. In practice, therefore, it can be assumed that the future role of compliance experts will be less about detection and more about the tackling of issues like for example internal processes optimization.
Making decisions about which processes AI can best help with
When it comes to using machine learning for compliance purposes, one of the first things to consider is which processes are most ripe for automation. IBM and Chartis’ research paper on Demystifying Artificial Intelligence in Risk and Compliance lists some of the factors that should be considered - primarily whether the process involves structured and recurring decisions.
For example, during the onboarding process in a bank or FinTech, there are a number of checks which need to take place before an account is created, and it's important here to strike a balance between risk-management and efficiency. Such processes are perfect for ML, as they enable ‘learning’ through repetition. On the flipside, ML isn’t suitable for low-frequency, rare or unusual events.
Should you adopt supervised or unsupervised AI?
As previously mentioned, there are different types of AI applications with varying degrees of human involvement. Financial institutions are strongly regulated, which means that management teams are reluctant to leave ML to make any significant decisions. As such, it makes much more sense for ML models to work as sorting mechanisms, with a human check still being made at the end of the process.
The importance of having the right data and data tools
Transaction monitoring requires financial institutions to analyse vast amounts of unstructured data from a range of sources. The more varied the different formats, the more challenging it is to work through the information and to detect false positives accurately.
The first step towards implementing an AI solution is to prepare your data. This might mean structuring and organising your data so that it’s all in the same format. This is particularly important when it comes to client names and other personal user data. A classic method used by many criminals is changing their name or moving between countries to try and thwart AML controls.
While working towards structuring your data is always a good idea, there are currently many market solutions that enable the processing of unstructured data, such as video, text and audio. These are particularly useful for fraud and financial crime cases requiring supporting information to enable financial bodies to make decisions. The most popularly used are NoSQL and Hadoop.
Conclusion
AI is already playing a significant role in improving the safety and efficiency of compliance processes. As technology advances and becomes more sophisticated, machine learning solutions are able to learn new patterns quickly. This therefore means that the focus of compliance professionals can be where it matters most - at the forefront of managing the whole process.
Want to speak to an experienced Machine Learning Team about your product?
Contact us today on +48 790 667 006 or hello@10clouds.com and our dedicated team will get back to you within one business day.