How Machine Learning can Drive the Efficiency of your FinTech Product
30.06.2021 | 6 min read
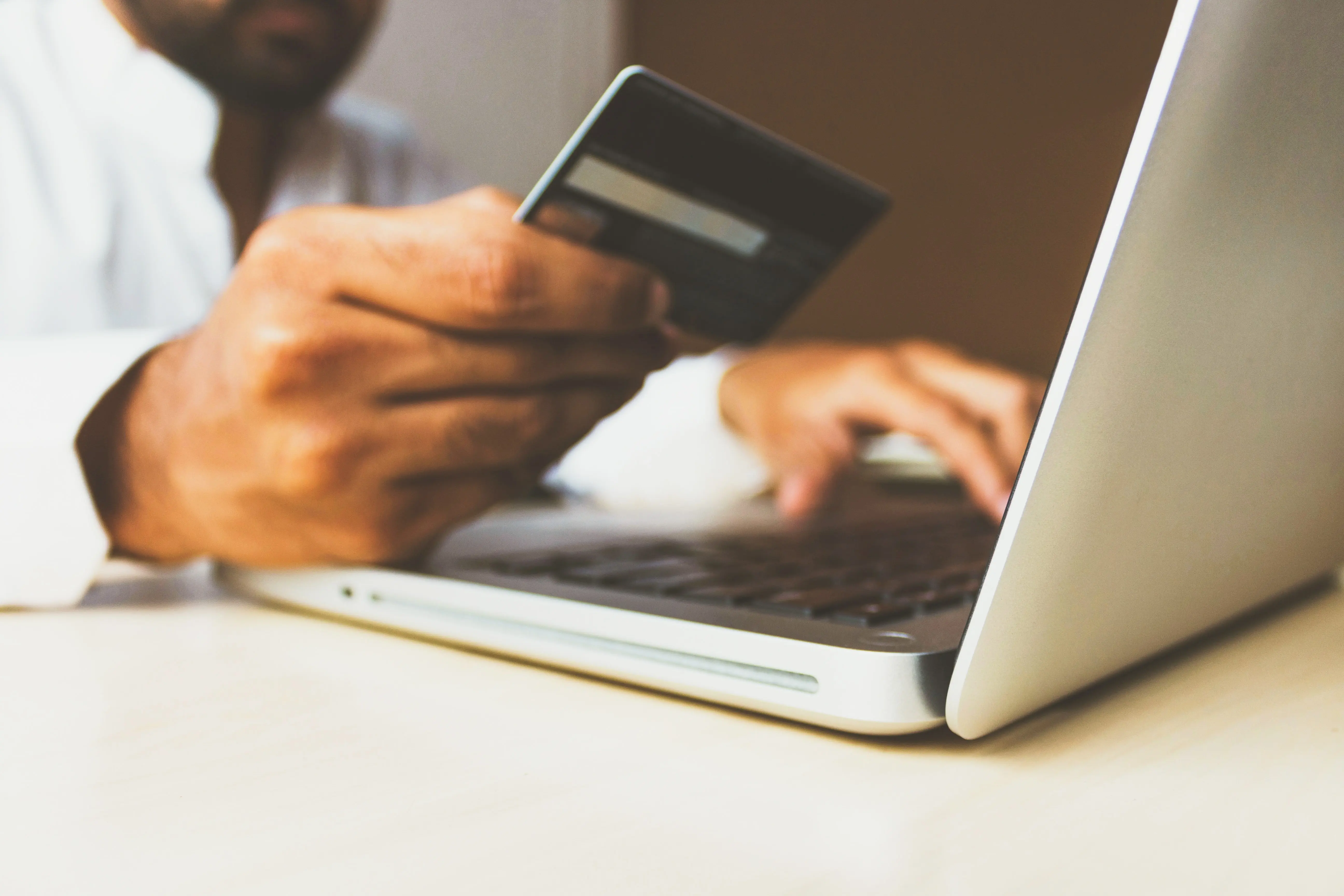
We recently wrote about the many benefits that Machine Learning can bring to businesses, mainly when it comes to improving process efficiency. According to a recent study, the most frequent use cases of AI and ML include reducing costs (38%), offering deeper customer insights (37%), automating internal processes (30%) and fraud detection (27%).
With a deeper understanding of customers’ needs, companies are able to optimize their market offering and consequently drive sales. And what better industry in which to put ML solutions to good use than FinTech and banking which is undergoing its own revolution in recent years? Let’s take a closer look at the specific ways in which ML can be put to use here.
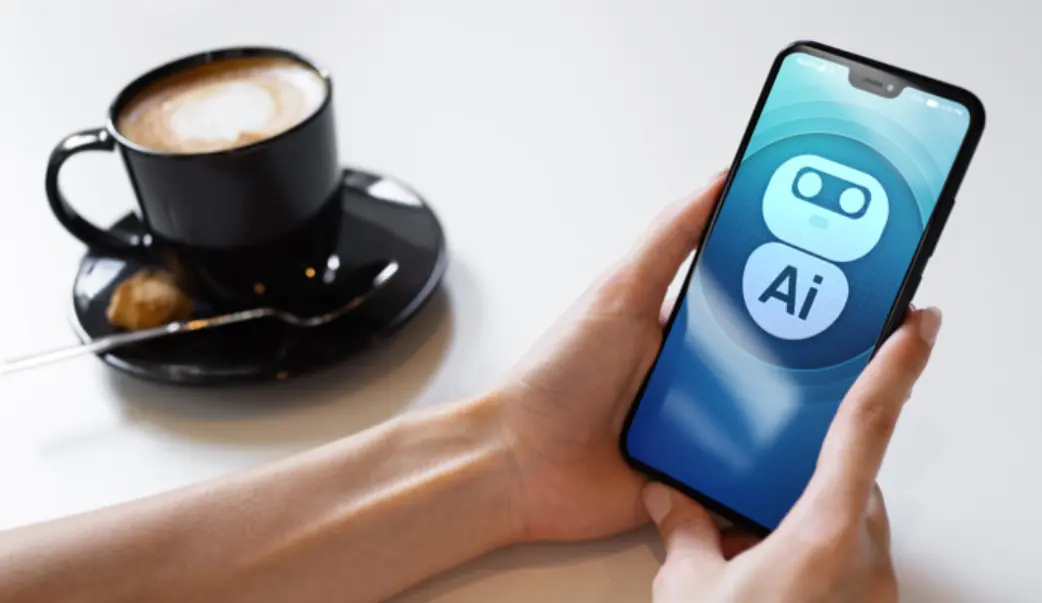
High quality customer service
When we look at the FinTech trends for 2021, we see a rise in digital-only banks. Covid had brought about the total eradication of the physical processes involved in banking. In this new age, customers have not only come to expect that all the usual functions involved with opening an account, or managing your money can be done online, but they also expect excellent customer service.
Chatbots: Studies have shown that 90% of customers want an immediate response to their question, and chatbots are able to deal with their queries quicker than ever before. The great news is that the technology not only analyses their enquiries, but also gathers useful data about customer needs.
Personalized solutions: As well as chatbot data, machine learning algorithms can be used to track user behaviour, finding out what matters to particular customers and creating personalised offers, removing the need for intervention from a customer service assistant.
Reduced risks for approving loans
Peer-to-peer lending is an area which has grown exponentially in recent years. It works by matching borrowers with lenders via online platforms or offline brokers. Peer to peer lenders and traditional banks alike, hugely benefit from effective credit scores. A machine learning credit-risk model takes the historical data of a prospective borrower, and uses that data, alongside the data of many other borrowers, to show how likely it is that a given borrower will be able to pay back the loan that they are requesting.
This is equally important for legacy lenders. These companies might benefit from ML and AI by limiting manual work and improving their predictions regarding different clients' risks. In many cases in traditional financial institutions evaluation models are based on the data from the far past (e.g. up to 3 years in corporate banking), which might be debatable from an accuracy perspective.
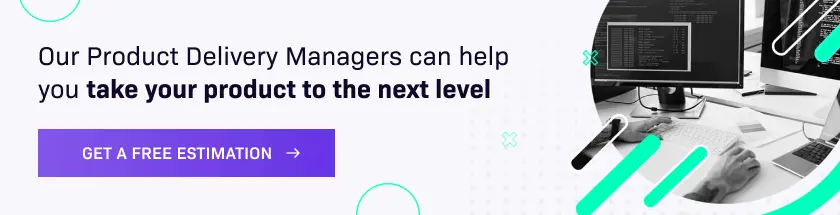
There are many types of data processed by credit-risk ML models, including income data, past credit scores, rent payments, utility payments and much more. Algorithms compare the aggregated data points with the data stored for thousands of other candidates in order to come up with as accurate a risk score as possible. A threshold is then set and if the score is below that threshold, the loan is automatically approved with no need for intervention from a customer service advisor. The result is fewer lending risks and more trustworthy credit scores. It also means that users can access credit much faster, thereby feeding into a better customer experience (as mentioned in the point above).
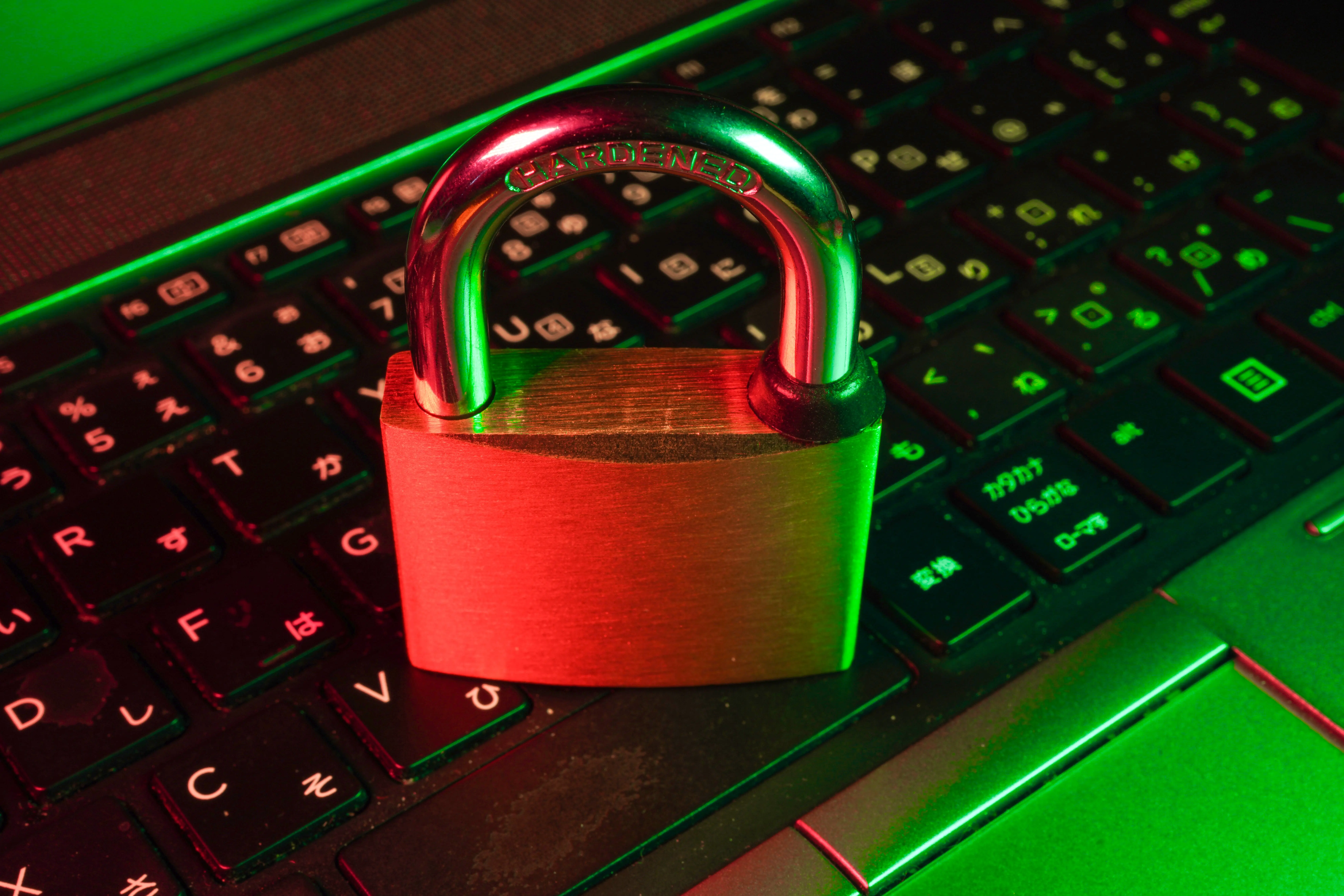
Fighting fraud
Fraud is an age-old and complex issue which has plagued banking institutions for decades and now is impacting FinTechs as well. With consistently increased digitization across the sector, hackers are finding and exploiting new weaknesses within digital products. What’s even more worrying is that fraudulent actors are sharing data and techniques, making systems even more open to vulnerabilities. So can machine learning really hold the answer? Well, it can certainly help. And here’s how.
Data analytics: As previously mentioned, ML algorithms have the capacity to analyze huge amounts of data in real time. This analysis includes the customer’s location, the device that they’ve used, and other data points which offer a much more holistic view of transactions. This functionality leads to faster decisions when it comes to fraud detection.
Attack detection: Following on from the point above, because ML algorithms have access to such vast quantities of data at any given moment, they are significantly better than humans when it comes to detecting new and emerging fraud attacks. And with time, these models will only increase in efficiency and accuracy.
Support in achieving regulatory compliance: The use of ML (and all the data that it analyzes), means that a bank or FinTech can regularly update its policies in line with changing needs, which is important when trying to maintain regulatory compliance over time.
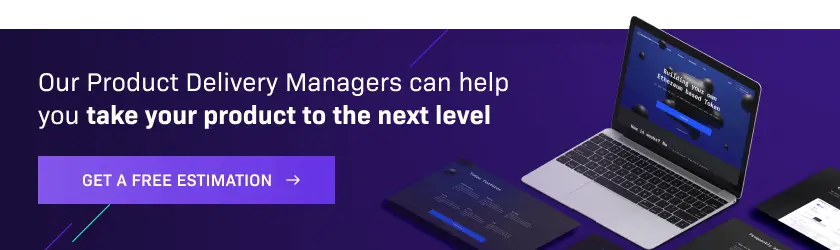
Stock market forecasting
Machine Learning algorithms are definitely better predictors of the future of the stock market than any trader or investor. The sheer quantities of historical data, and the fact that they can monitor data in real time, means that highly accurate predictions can be made about the future. ML can detect even slight shifts in the price of stock, so it is down to traders to react accordingly and to select which algorithms they want to use in their strategy. An additional benefit to using ML in this field is the fact that it removes the bias associated with human analysis.
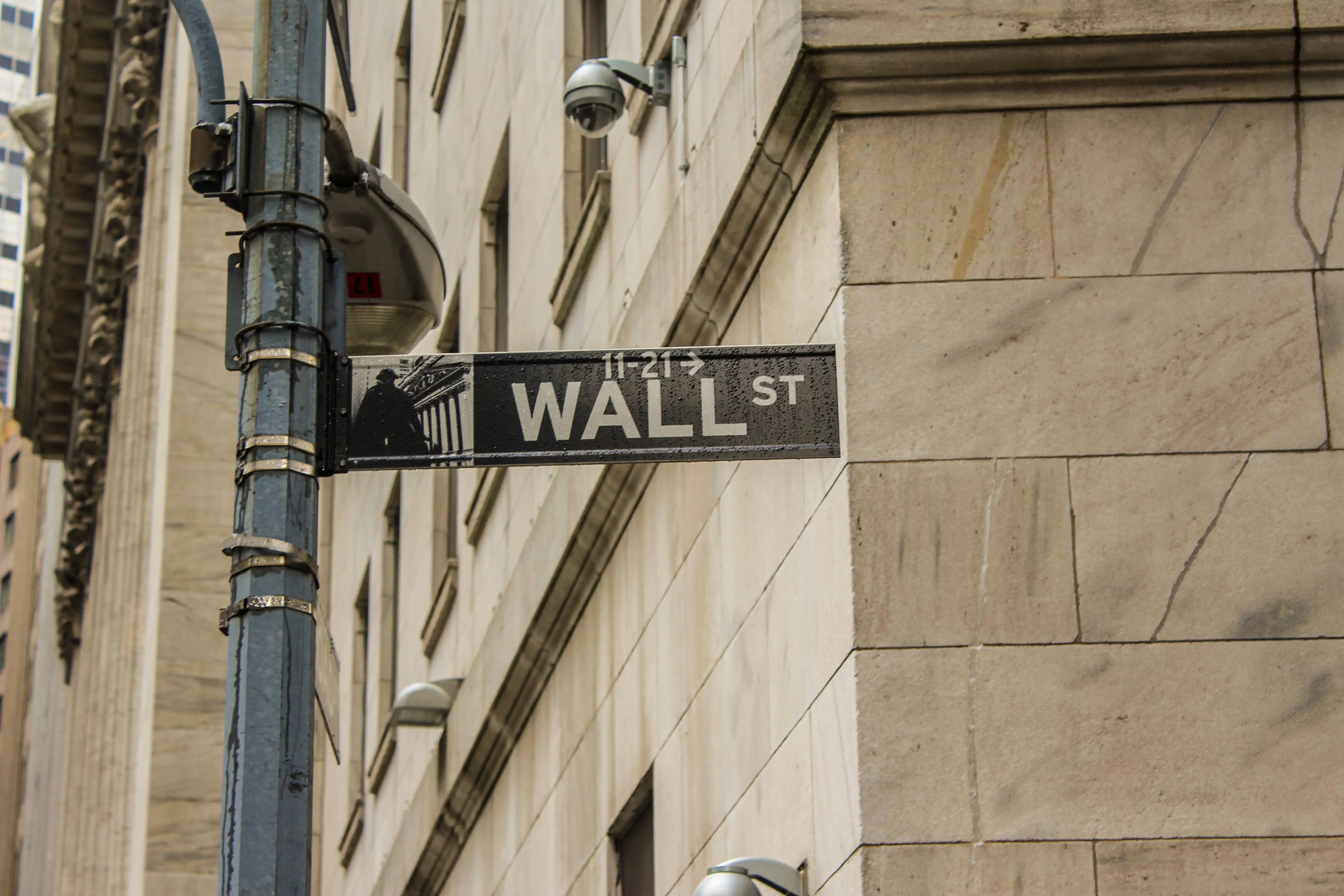
Robo-advisors
Robo-advisors have been growing in popularity in recent years. They are digital platforms providing ML-driven financial planning services with very little human interaction. Typically, they collect information from clients about their financial situation through the use of surveys. They then use the data that they collect to automatically invest client funds.
There have been some questions raised about both the accuracy and the ethics of robo-advisors, but they are consistently being refined to produce better results. Over the past year or so, we’ve seen a gradual shift from the previously-mentioned surveys to automated, self-learning algorithms. The newest solutions in this sphere recommend investment opportunities based on investment habits and risk appetite as well as income.
But which ML solution is right for your banking or FinTech product?
While many C-Level Execs understand that ML can benefit their product, they are unsure of where to start. Our advice would be to begin by looking at your USP. If your primary goal is to deliver a fast, seamless digital experience for your banking clients, consider the implementation of a tailored chatbot. If your focus is more around the elimination of risk and the desire to guarantee the safest transactions on the market, consider building an ML model for fighting fraud and building this into your product. The key is to focus on one key element, rather than having a scattergun approach to ML.
Want to speak to an experienced Machine Learning Team about your product?
Contact us today on +48 790 667 006 or hello@10clouds.com and our friendly team will get back to you within one business day.