Examples of Projects in which You Should and Shouldn’t Use Machine Learning
02.04.2021 | 8 min read
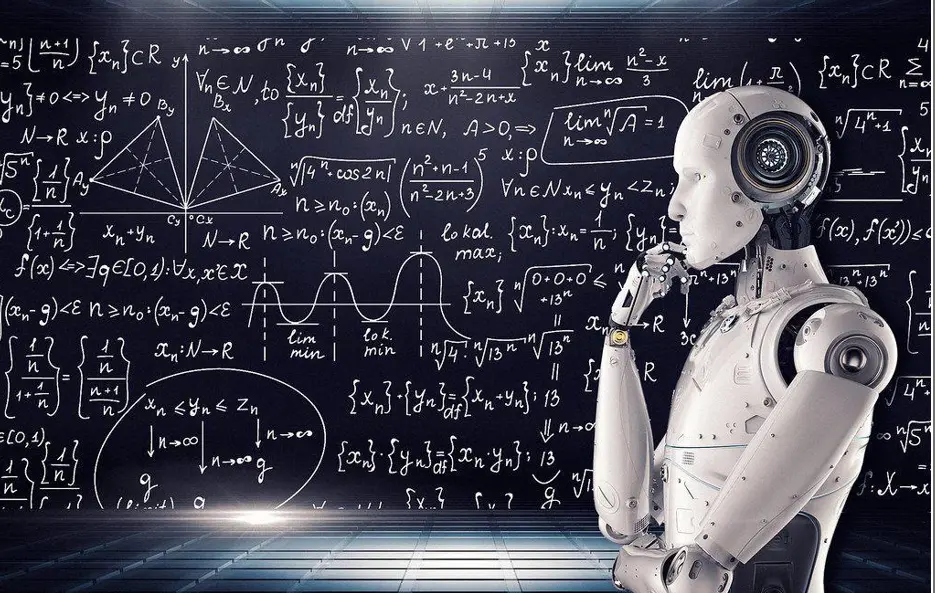
Ever since the emergence of machine learning as a concept, it has proved to be a powerful technique for predictive analysis. In the first section of this blog post, we will discuss the types of projects in which you should use machine learning. In the later section, we will focus on the projects in which using machine learning might not come up as the best option. For both of the above-defined scenarios, we will reflect on some example projects to better understand the purpose.
Machine learning and today’s world
Ever wondered how Facebook shows you videos in the feed similar to what you have already watched before? Machine learning, today, is a buzzword and is spread among everyone who is concerned about the future of technology.
A major chunk of technological innovations that involve automation are either directly using machine learning as the core infrastructure or are somehow influenced by it.
The day-to-day interactions, decisions, influences, are all governed by this idea. A simple example in order to understand it will be recommendations systems. We encounter recommendation systems every day in our lives; watching movies on Netflix, purchasing stuff from Amazon, etc. All these applications internally make use of machine learning models to capture users’ preferences and hence treat us based on the relevance of choices.
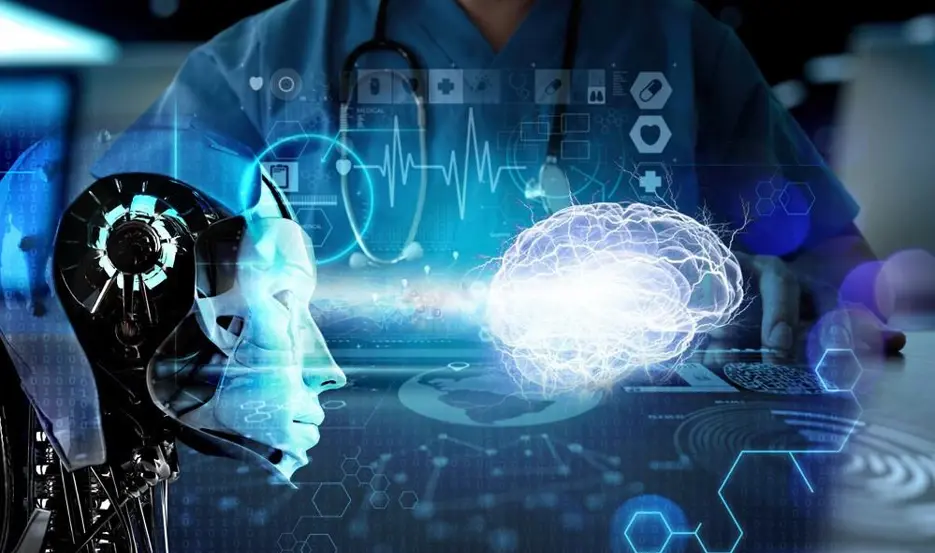
Why machine learning?
We are living in an agile world where the thought process one might have today could completely contradict what was yesterday. This is due to the changing needs, demands, and practices in society. Everyone is contributing to society in one way or the other. As a result of an individual’s contribution, the behaviors and practices of society are shaped.
Amidst all this, everyone is consciously or unconsciously producing data which is the biggest game-changer in today’s world. Just during the last decade, we have produced tons and tons of data. In order to keep up with the pace of this technology-driven ecosystem, we must utilize the power of machine learning to comprehend the insights and relevant outcomes caused as a result of this data.
Eventually, at the end of the day, the data that we produce dictates certain trends within the global society so we can enable machine learning to give us concrete action items to further solidify its applications as per the expectations.
Projects in which ML should be used
Following are some of the domains in which enterprises are actively focusing on using ML and rightly so.
Healthcare
With every advancement in the field of medical science, comes a huge challenge of appropriately locating the medical problems and effectively put forward their resolutions with a wise sense of maturity and responsibility. When it comes to healthcare analysis, machine learning can play a vital role in automating complex processes and decisions that would save a lot of doctor’s manual efforts and time.
Some of the really cool and fascinating machine learning applications in healthcare could be as follows.
- Automatic identification of diseases
- Accurately identifying bone damages in X-ray
- Prediction of medicines (given the disease parameters)
- Prediction of future disease and discovery
These are some of the very basic applications in which machine learning can be applied with respect to healthcare analysis. In fact, there are already some inspirational companies that are using ML for a similar purpose. You can read more about those companies and their work in this informatory article.
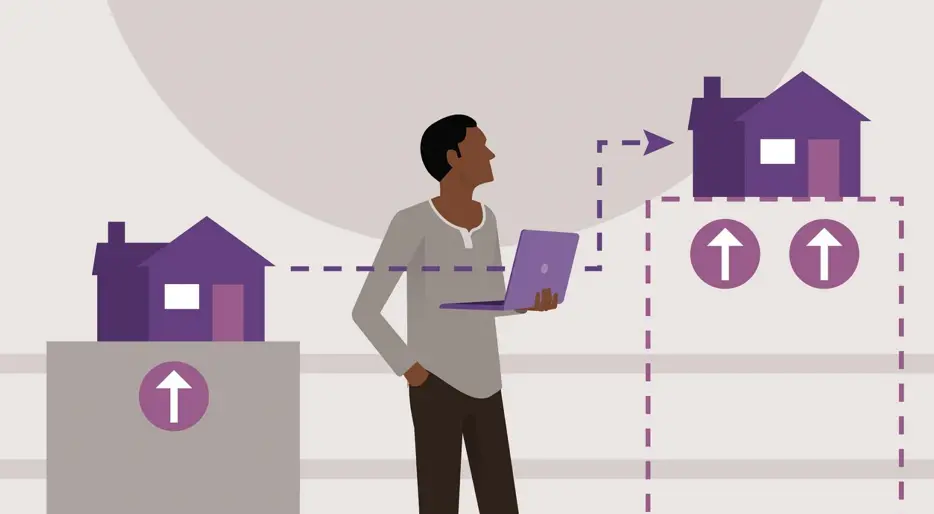
Recommendation systems
Recommendation systems are by far one of the most common and interesting applications that ML has ever addressed. It can be used in an enormous number of ways. To simplify, every application which is dependent on user choice can be automated using machine learning. If you are developing an application platform and want to transform the experience of it differently for every user then you must use ML-based recommendation systems in order to give your users a personalized sense of navigation experience.
Following are a few projects where you can use recommendation systems (machine learning) to diversify the application experience.
- Online shopping applications
- Video streaming platforms (similar to YouTube and Netflix)
- Audio distribution platforms (similar to SoundCloud, Google Play Music)
- Online learning experiences
- Social media applications, and much more
Content spam
“We are an XYZ company and pioneers of ABC product. Click on the following link to know more!” Ever received these spam marketing emails? They are just too annoying sometimes. Prevention of content spam is a popular area where machine learning has started growing its roots. You can build an interesting project that helps you filter spam emails (not just marketing ones but others as well) and as a result, only emails that are relevant to your purpose can reside inside your mailbox and that way you can have a better digital experience.
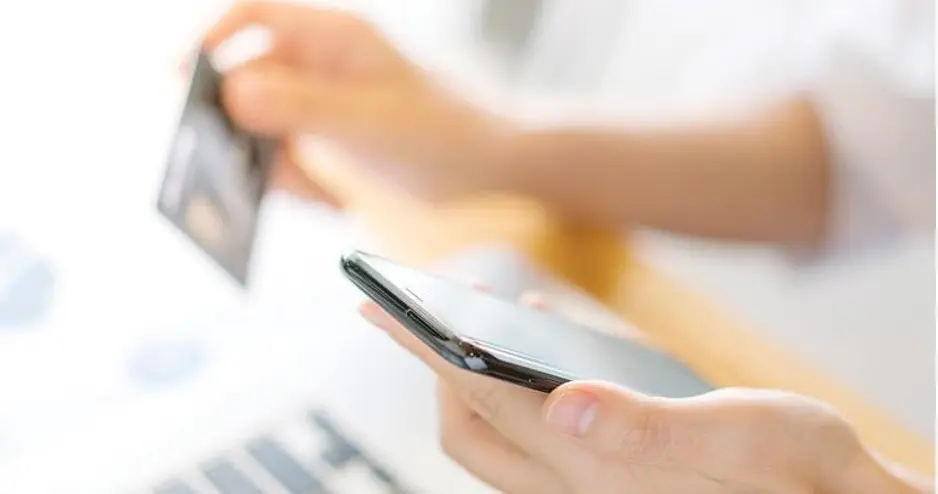
Financial technology
FinTech is one of the fastest emerging technologies out there. It has revolutionized the way traditional banking used to operate. It is an emerging technology that focuses on financial inclusion. With the advent of FinTech, there are a lot of scenarios in which we can take help from machine learning in order to bring a change. AML (Anti Money Laundering), fraud detection, consumer market trends, credit risk assessments are some of the key areas for which we can rely on ML to do us the wonders. Here is the list of some already built ML applications in the domain of financial technology.
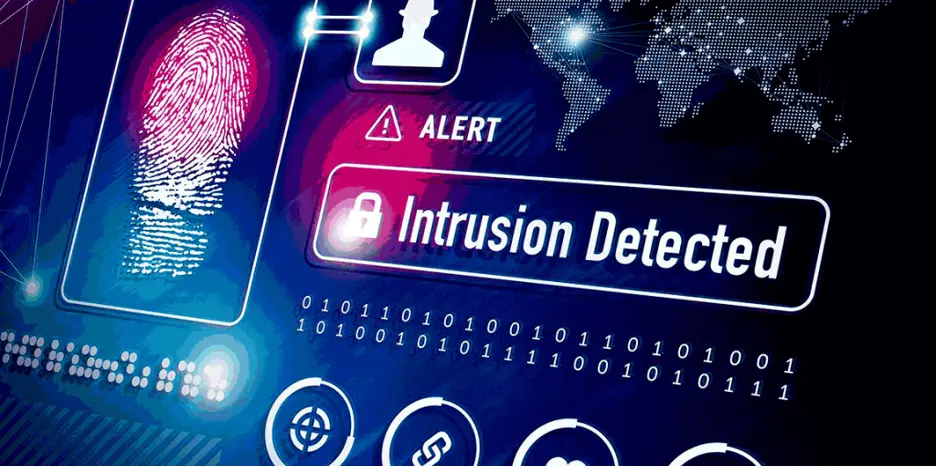
Detection systems
There are various types of detection systems from almost every walk of life. Be it in healthcare, e-commerce, social media, you name it! A few of the fascinating projects that you can pull off with the “detection” domain can revolve around facial detection or facial recognition, audio recognition, anomaly detection, etc. One cool viewpoint would be to deploy a facial recognition system within your company to mark the attendance of employees automatically instead of them swapping their cards or registering their fingerprints.
The above-discussed cool ML domains and projects are just some of the breathtaking wonders that ML has produced. The field is not limited to them but can be extended to various other application paradigms such as online trading, the internet of things, cybersecurity, e-commerce, language translation, and much more.
Have you noticed a trend while we were discussing the above-mentioned applications? In all the areas where ML is serving as a deal-breaker, they produce millions of data of points and hence empower the machine learning models with more and more data each day; because at the end of the day, the larger the dataset, the more accurate the results will be!
With that note in mind, let’s proceed towards the other part of the story.
Machine learning as a misunderstood concept
By far, we have looked and reflected on the outstanding applications that ML can serve but often times the concept of machine learning is misinterpreted. We tend to think of it in terms of the revolutionary outcomes that it can produce but it really depends on the use cases and the nature of problems it tries to solve.
We need to understand that although machine learning solves various complex problems, it is indeed not the solution to every problem that we face. Sometimes, it is overkill to apply ML techniques to problems that could be solved otherwise using simpler and straightforward techniques.
Projects in which ML shouldn’t be used
By now, you must have realized that the pain points in which you can actually capture a large amount of data are ideal situations where we can confidently deploy machine learning strategies for the better good. This alternately means that when you don’t have enough data for your problem then it is not at all recommended to train ML models. This has a very simple reason; if you don’t have much data then your model will not be able to accurately capture the insights from the data you provided and it will most likely overfit on the test dataset. So be very sure that if you do not have supporting data, reconsider your decision of choosing ML and figure out some other strategy that could help you find a relevant solution.
Another concerning point is the scenarios in which you have got less time to deliver your resolution. This means if you are interested in figuring out solutions to problems that are worthwhile for a short period of time then you should probably not use machine learning. Alternatively, you can use multiple heuristic-based approaches that can help you achieve similar results.
Machine learning models are generally known for accurate predictions over a comfortable period of time and if your project needs a rush, you should try something else as experimentation with models and later the training of ML models take time.
Lesser data points and shorter project timelines are the implementation barriers that prevent us from using machine learning but there is one major aspect known as a target market as well. If you know your target market, well and good! But if you are not familiar with your target market, then no matter how good your ML model is, there is no way to gauge the performance of your ML model on the sample population. Hence it is extremely necessary to opt for machine learning if you confidently know your target market and the population you are going to experiment on.
Conclusion
We hope by now you have developed some intuition as to where to opt for the wonders of machine learning and where not to! This was all from this blog post, let’s wait and watch what the researchers have to say about further exceptional advancements in the field of machine learning in upcoming years!